“Next Gen” Spotlight: Tahsin Alamgir Kheya
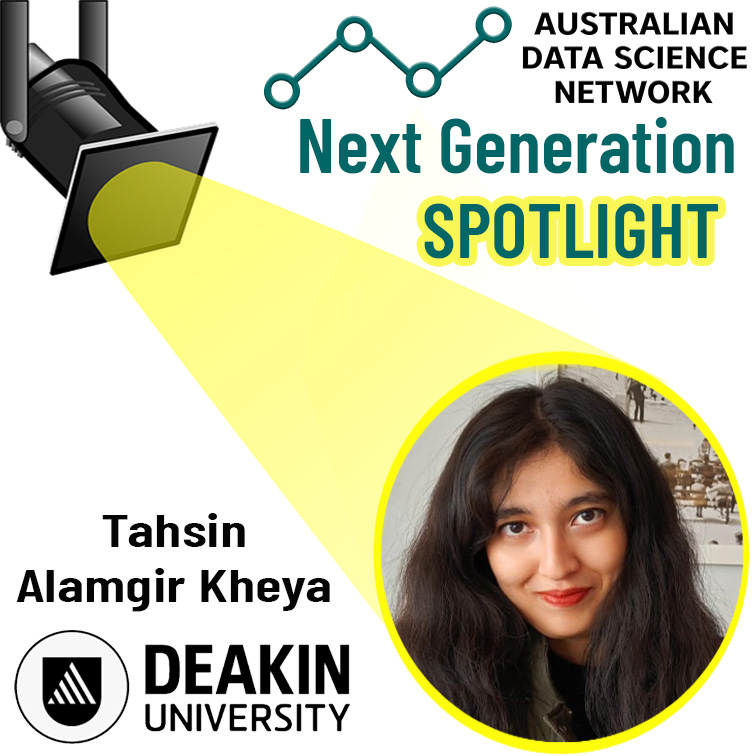
Tell us a bit about yourself
My name is Tahsin, and I am a first-year PhD candidate at Deakin University. I obtained my Bachelor's degree with honors in Software Engineering from Monash University. My current research focuses on evaluating and mitigating bias in Artificial Intelligence (AI) models, with the goal of developing tools to detect and address bias in real-world applications. From my standpoint, technology should be equitable and inclusive, and I am enthusiastic about contributing to this area to help make that vision a reality.
What is your PhD in and why?
I am undertaking my PhD under the guidance of Dr.Sunil Aryal and Dr.Mohamed Reda Bouadjenek. My work seeks to contribute to the field of fair recommendation systems, with a special focus on social bias. Recommendation systems help users by curating items according to their preferences, that not only helps reduce information overload for them but also enhances their experience. Amid the potential of such systems however, concerns about the manifestation of bias in them arise. Biases in these models can lead to unexpected discriminatory practices like partiality for certain genders, ethnicities, or disabled people.
I intend to explore diverse approaches to effectively evaluate and mitigate bias in such systems. Additionally, I aim to highlight the importance of investigating the implications of issues like filter bubbles and bias to ensure a more ethical approach in designing AI models. Ultimately, my research aims to spread awareness of bias in recommender systems, provide comprehensive ways to evaluate bias in them and mitigate them by looking at the issue at a granular level. By working on these aspects, I want to achieve models that promote equitable recommendations to different individuals and groups.
Any surprises or highlights from your PhD?
Throughout my first year of PhD, I was surprised to see how much my research direction evolved over time. Initially I expected to stick to my plan of using reinforcement learning for mitigating bias in AI models. But new discoveries and feedback from my advisors led me to explore unexpected areas that I hadn't originally considered. While it was challenging, it was also exciting to adapt and dive into new topics.
How did you get interested in Data Science?
While undertaking my studies in Monash, I was introduced to data science through several compulsory units. These units focused on the analysis of big data and sparked my deep interest in data science. They introduced techniques like data pre-processing, management, transformation and visual analysis. Using these methods helped me see the power of data to uncover patterns and insights across diverse case studies. I also got to investigate real-world datasets and developed a critical understanding of how data is analyzed and how these techniques can apply to various fields. I also realized its applications to AI model training, where clean and well-structured data can help achieve accurate results.
What do you see as the big challenge facing the ADSN and the entire Data Science Community? Is there a big research question we should be tackling?
One challenge faced by the data science community is ensuring that the models deployed to be used by the society are trained and designed in a way that aligns with ethical principles and societal needs. Issues like fairness, transparency, interpretability and privacy are concerns that need highlighting. While these are already being researched on actively, one research question that we should try to address is: How to ensure data-driven systems that are used across various domains (including high-stakes areas like healthcare, criminal justice system) are interpretable and fair for every individual and group in the society. We should aim to ensure that insights derived from data science serve the public good.
Fun fact about yourself.
As a researcher working on bias in AI, it is ironic how I spend my time teaching models to not “judge” based on certain attributes, yet here I am, judging them constantly.